Description:
Magnetic Resonance Imaging (MRI) is the most commonly used imaging modality for diagnosis and surveillance of various neurosurgical conditions, while patients usually need to undergo a rigorous screening process prior to MRI examination to ensure the implants they have are MR safe and assure patient safety. Researchers at UTHealth have developed a new pipeline to identify implantable device MRI compatibility using mobile phone quality images, which outperforms the other currently available methods, and has the potential to become a safety tool for radiologists to efficiently coordinate the care of patients with implanted devices.
Technology Overview
Accurate information concerning implanted medical devices prior to an MRI examination is crucial to assure the safety of patients and to address MRI-induced unintended changes in device settings. Researchers at UTHealth have developed a deep learning enabled automatic system for implanted medical devices MRI compatibility determination using X-ray images of the radiologist workstation screens captured with mobile phone integrated cameras at different angles and illuminations. Compared to other methods, this shows several advantages:
-
- This automatic identification system can greatly accelerate the whole process for pre-MRI screening and save time for both patients and radiologists
- The pipeline can be easily integrated into the hospital workflow as it does not require any software installation in the hospital workstations or PACS
- The images captured with the mobile phones do not have any metadata containing protected health information (PHI), thereby drastically reducing security concerns in the translation of the project to clinical practice.
Pipeline of the implantable medical devices MRI compatibility identification framework. Mobile phone application is integrated as part of the PACS
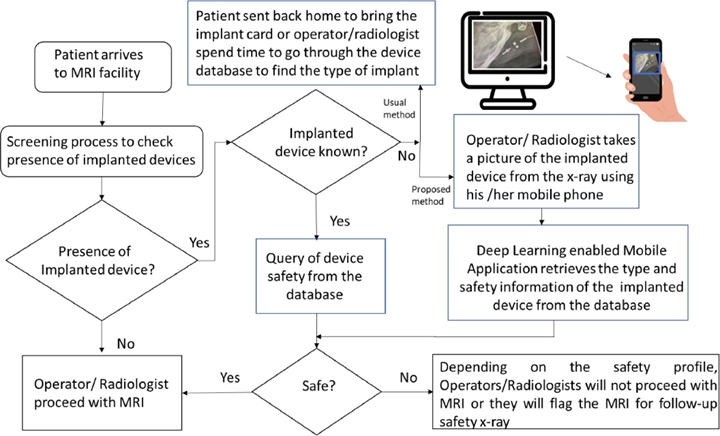
Associated Publications
Comput Methods Programs Biomed. 2021 Oct; 210:106356. doi:10.1016/j.cmpb.2021.106356
Sci Rep. 2018 Oct 30; 8(1):16052. doi: 10.1038/s41598-018-34164-6
About the Inventors
Dr. Eliana E. Bonfante-Mejia, MD: Clinical Associate Professor, Department of Diagnostic And Interventional Imaging at UTHealth
Dr. Luca Giancardo, PhD: Assistant Professor at the Center for Precision Health, School of Biomedical Informatics (SBMI); co-appointments at McGovern Medical School and the Institute for Stroke and Cerebrovascular Diseases, UTHealth
Dr. Roy F. Riascos-Castaneda, MD: Professor, Department of Diagnostic and Interventional Imaging at UTHealth
UTHealth Ref. No.: 2018-0035